Recent advances in Large Language Models have driven large-scale deployment, resulting in ever-growing
inference time and energy demand. While manual optimization of low-level code implementations is
feasible, it is an arduous task that requires deep expertise to balance the complex interplay of
algorithmic, software, and hardware bottlenecks. We present The AI CUDA Engineer, the first
comprehensive agentic framework
for fully automatic CUDA kernel discovery and optimization, enabling frontier large language models to
perform the translation of torch
code to CUDA kernels and then iteratively improve their runtime.
The AI CUDA Engineer can robustly translate PyTorch operations to CUDA (>90% success rate), and optimize these operations better than native torch (∼75% success rate) or even torch compile (∼60% success rate). For certain operations, such as Instance Normalization and lower triangular matrix multiplication, we demonstrate remarkable speedups of up to 381x and 147x, respectively.
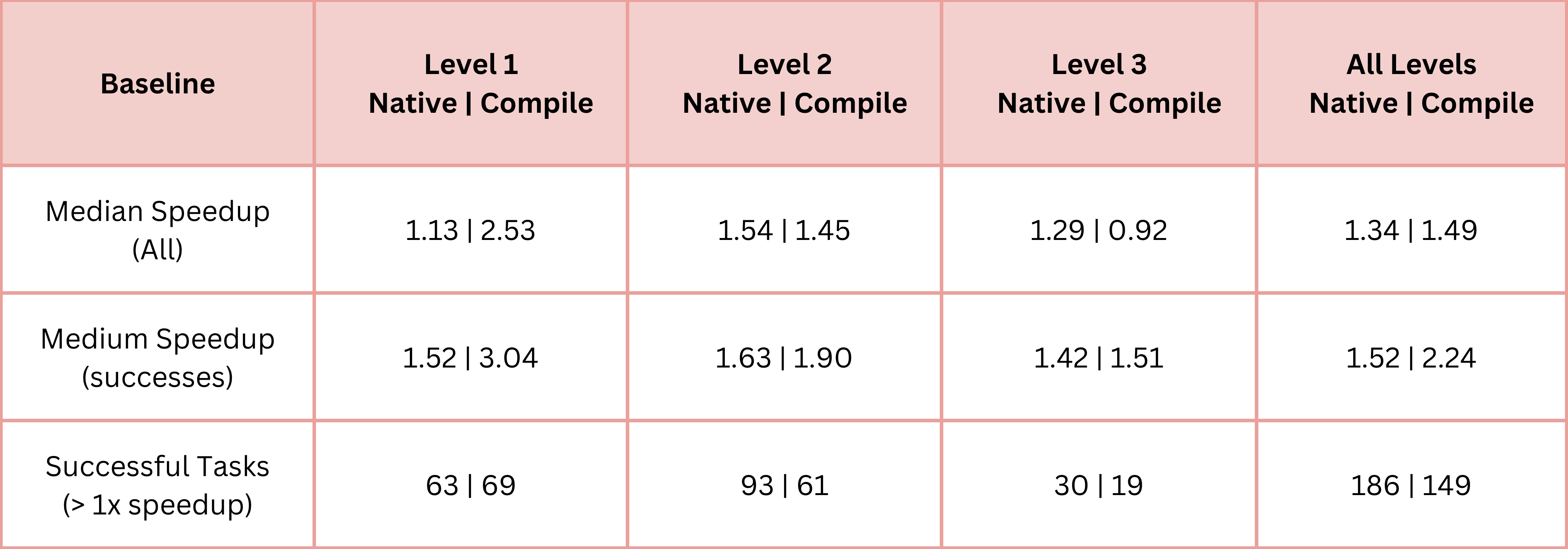
The AI CUDA Engineer reaches a 1.34x median speedup over torch native across all 250 tasks, and a 1.52x speedup over the 186 successful tasks.
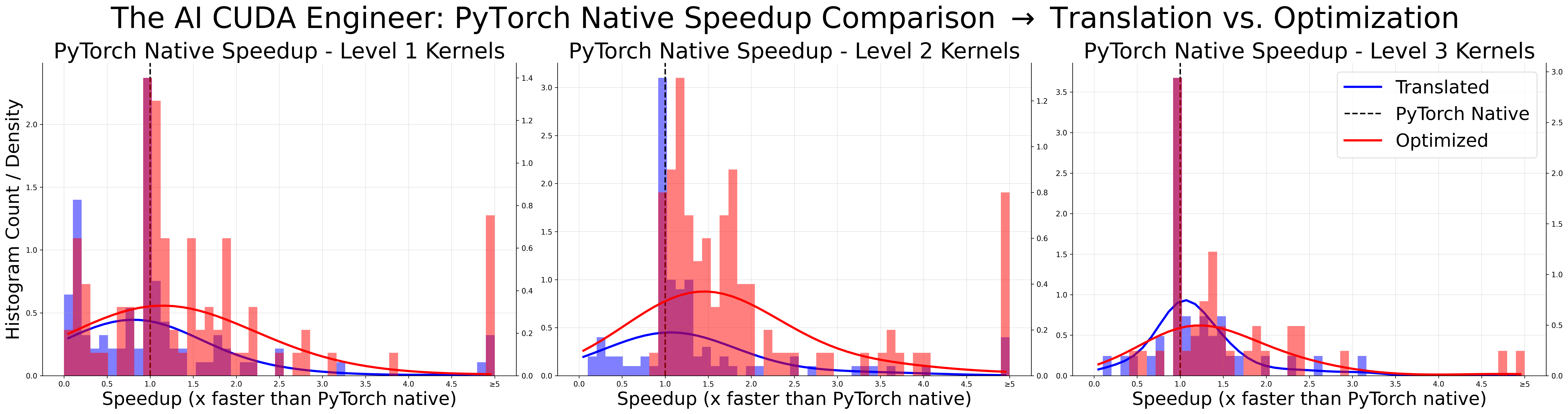
The AI CUDA Engineer Archive: A Verified CUDA Kernel Dataset
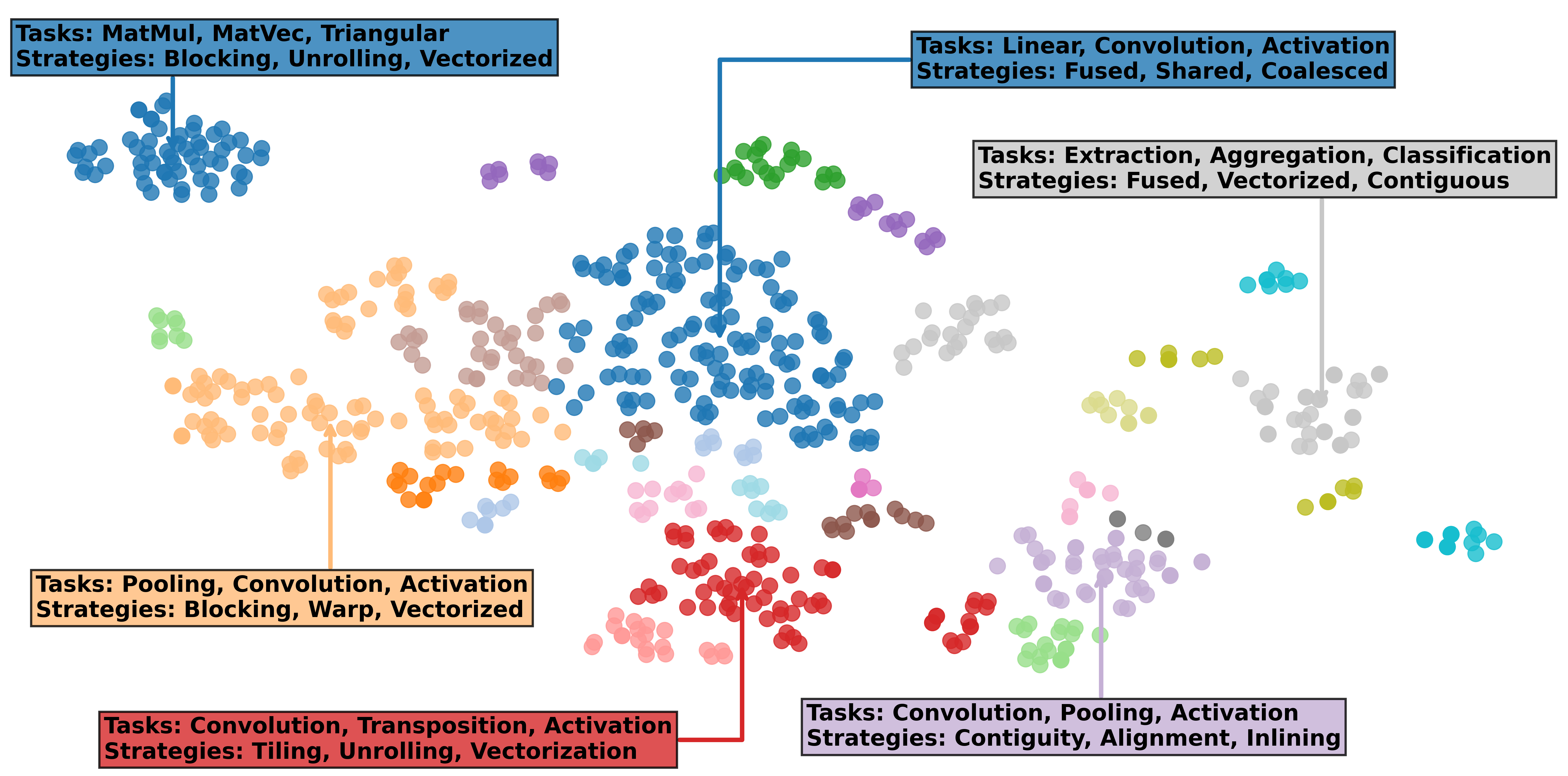
Along with this paper, we release the AI CUDA Engineer archive, a dataset consisting of approximately
30,000
CUDA kernels generated by the AI CUDA Engineer. It is released under the CC-By-4.0
license
and can
be accessed via HuggingFace and
interactively visualized here. The dataset includes a
torch
reference implementation, torch
, NCU
and
Clang-tidy
profiling data, multiple kernels per task, error messages and speedup scores
against torch
native and compile runtimes.
We envision that this dataset can enable post-training of open-source models to perform better
CUDA-enabling modules. This includes offline Reinforcement Learning, preference optimization, and
standard supervised fine-tuning.
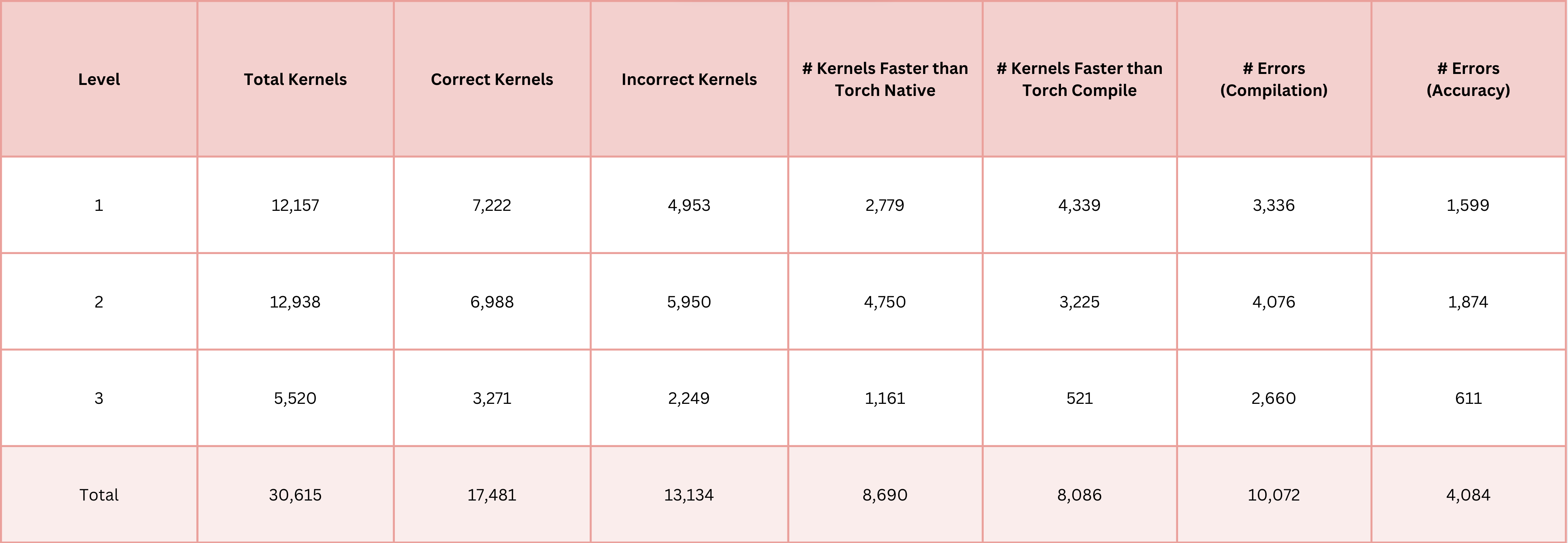
Citing The AI CUDA Engineer & Archive
If you use the AI CUDA Engineer or the archive in your work, please cite the following paper:
@article{lange2025aicudaengineer,
title = {The AI CUDA Engineer: Agentic CUDA Kernel Discovery,
Optimization and Composition},
author = {Lange, Robert Tjarko and
Prasad, Aaditya and
Sun, Qi and
Faldor, Maxence and
Tang, Yujin and
Ha, David},
journal = {arXiv preprint},
year = {2025}
}